We’re excited to announce that the AI City Challenge has been officially accepted as a workshop at ICCV 2025. Join us as we continue advancing computer vision and AI in real-world Settings.
2025 AI CITY CHALLENGE
The AI City Challenge, hosted at ICCV 2025, is designed to push the boundaries of computer vision and AI in real-world settings, driving innovative solutions for smarter transportation, large-scale industrial analytics, and public safety. By tackling multifaceted data sources—from cameras and lidars in roadway intersections
to high-fidelity synthetic warehouses—participants in this challenge will develop and benchmark new methods capable of gleaning actionable insights under real-time or near real-time constraints.
This 9th edition of the Challenge introduces exciting new tasks and significant dataset upgrades and provides four challenging tracks as below:
Challenge Track 1. Multi-Camera 3D Perception *: Teams are tasked with tracking individuals across a network of cameras. Compared to last year, a larger-scale synthetic dataset is created by NVIDIA Omniverse with more diverse object classes (people, autonomous mobile robots, humanoids and forklifts). 3D bounding box labels and detailed calibration are provided. Tracking is scored by HOTA (Higher Order Tracking Accuracy) [4], with a 10% bonus for online methods that use only past frames at inference.
Challenge Track 2. Traffic Safety Description and Analysis: Using multiple cameras and viewpoints, participants are challenged to describe both the moments leading up to incidents and the normal traffic flow, capturing all relevant details about pedestrian and vehicle behavior. The task also includes a video question-answering component to assess fine-grained understanding. The dataset has been enhanced with 3D gaze annotations and traffic video question answering. Quantitative scores will reflect accuracy on question answering, caption quality, and the fidelity of scene reconstruction in terms of directions, actions, and attributes.
Challenge Track 3. Warehouse Spatial Intelligence *: Modern warehouses are dynamic environments filled with diverse objects, changing layouts, and critical safety conditions. Understanding the spatial relationships between objects — such as measuring distances, assessing free space, and verifying alignment — is essential for effective warehouse management and automation. This challenge focuses on developing AI systems that can answer fine-grained spatial questions from RGB-D images, integrating visual perception, 3D geometric reasoning, and natural language understanding to improve operational intelligence in industrial settings.
Challenge Track 4. Road Object Detection in Fish-Eye Cameras: This challenge centers on road object detection with fisheye cameras, prioritizing resource efficiency and edge computing. Participants develop Docker containers for NVIDIA Jetson devices, with performance evaluated by the harmonic mean of F1 score and FPS to promote lightweight, real-time applications.
Participants are invited to compete in one or more of the five challenge tracks. To join, please navigate to the page of your target track(s) under CHALLENGE tab.
* The dataset was generated using the Isaacsim.Replicator.Agent (IRA) and Isaacsim.Replicator.Object (IRO) extensions in the NVIDIA Omniverse platform.
Important Dates
ICCV 2025 work shop date is not finalized yet and below is the tentative timeline for AI City Challenge 2025 compiling with current ICCV schedule.
-
Release of training and validation data sets: April 30, 2025 -
Release of evaluation server and test data sets: May 30, 2025 -
Challenge track submissions due: June 30, 2025 (6:00 PM, Pacific Time) -
Workshop papers due: July 06, 2025 (11:59 PM, Anywhere on Earth) - Acceptance notification: July 11, 2025
- Camera-ready papers due: August 18, 2025
- Open source on GitHub (training code + testing code + additional annotation) due: September 19, 2025 (6:00 PM, Pacific Time)
- Presentation of papers and announcement of awards: TBD
Workshop Committee
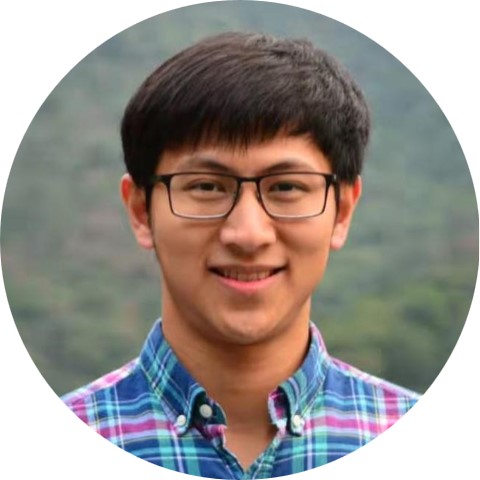
Zheng Tang
NVIDIA
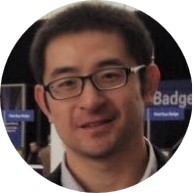
Shuo Wang
NVIDIA
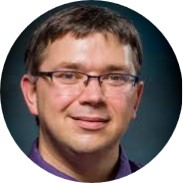
David Anastasiu
Santa Clara University
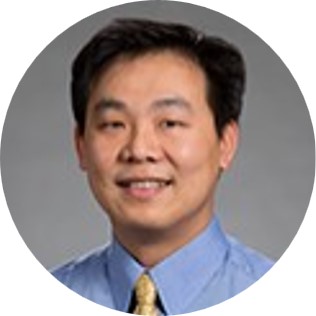
Ming-Ching Chang
University at Albany – SUNY
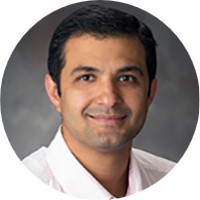
Anuj Sharma
Iowa State University
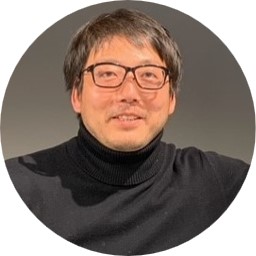
Norimasa Kobori
Woven by Toyota
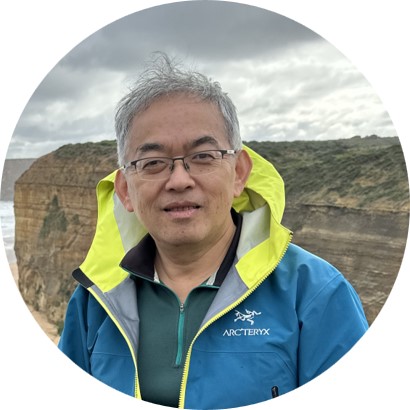
Jun-Wei Hsieh
National Yung-Ming Chiao-Tung University
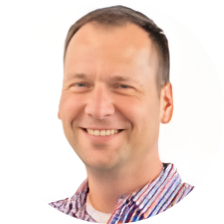
Tomasz Kornuta
NVIDIA
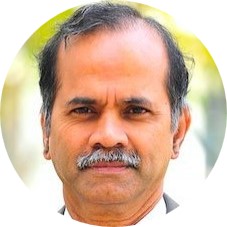
Rama Chellappa
Johns Hopkins University
Challenge Committee
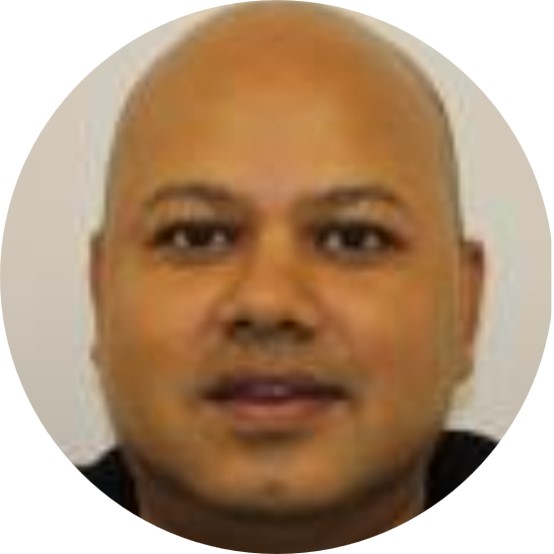
Sujit Biswas
NVIDIA
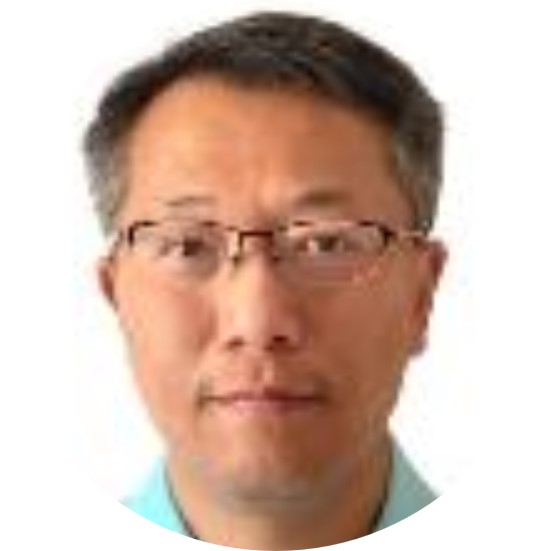
Xunlei Wu
NVIDIA
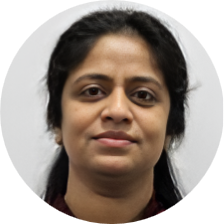
Vidya Murali
NVIDIA
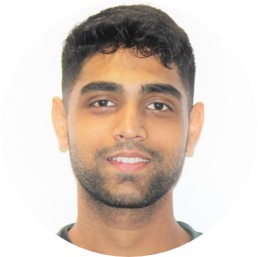
Sameer Satish Pusegaonkar
NVIDIA
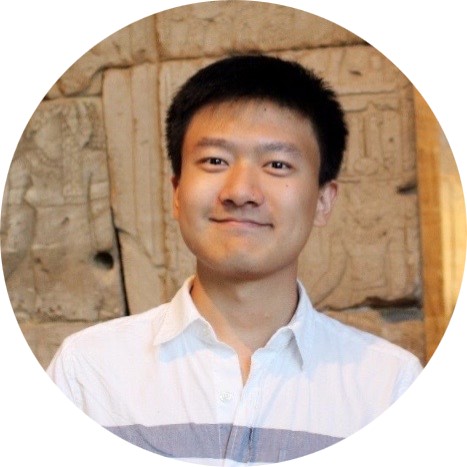
Yizhou Wang
NVIDIA
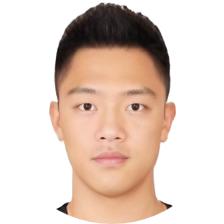
Yuxing Wang
NVIDIA
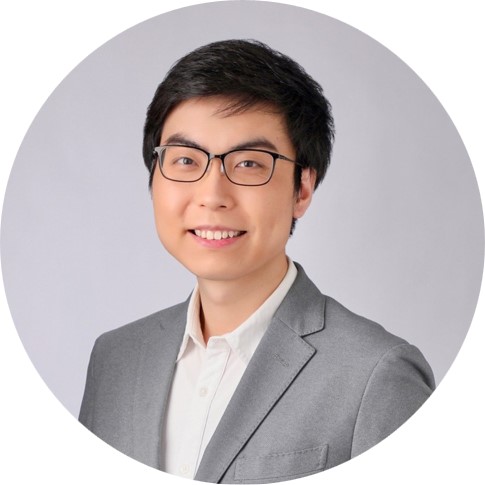
Quan Kong
Woven by Toyota
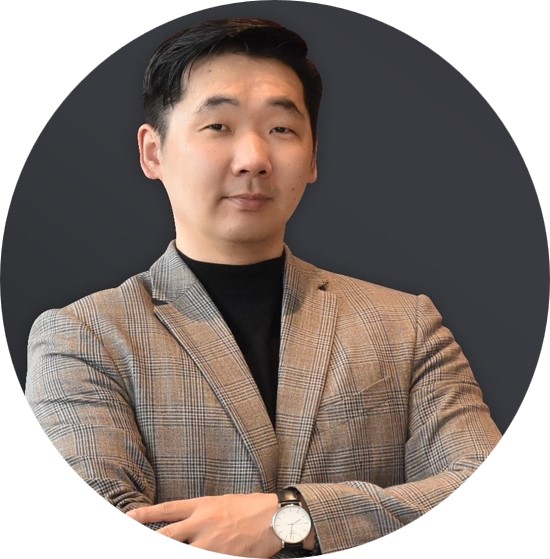
Munkhjargal Gochoo
The United Arab Emirates University
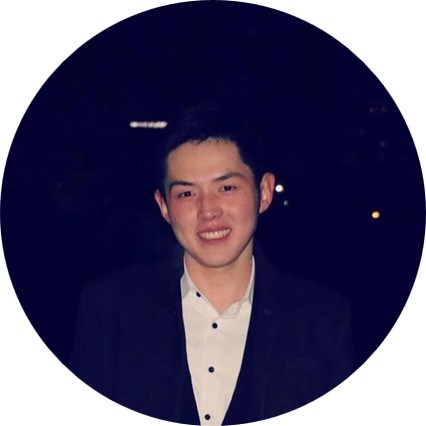
Ganzorig Batnasan
Mirai
Technologies
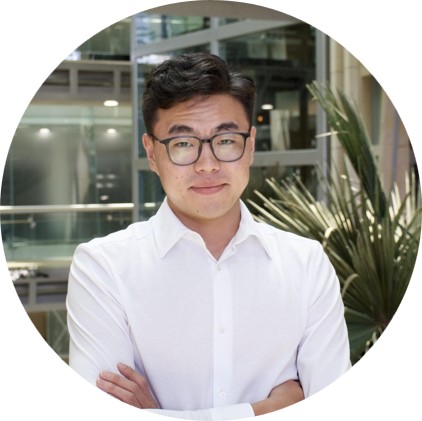
Munkh-Erdene Otgonbold
Mirai Technologies
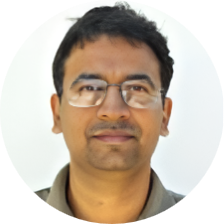
Ratnesh Kumar
NVIDIA
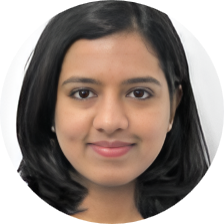
Subhashree Radhakrishnan
NVIDIA
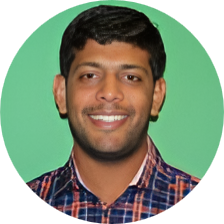
Arihant Jain
NVIDIA
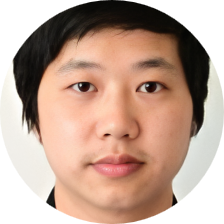
Xiaolong Li
NVIDIA
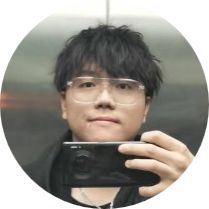
Yilin Zhao
NVIDIA
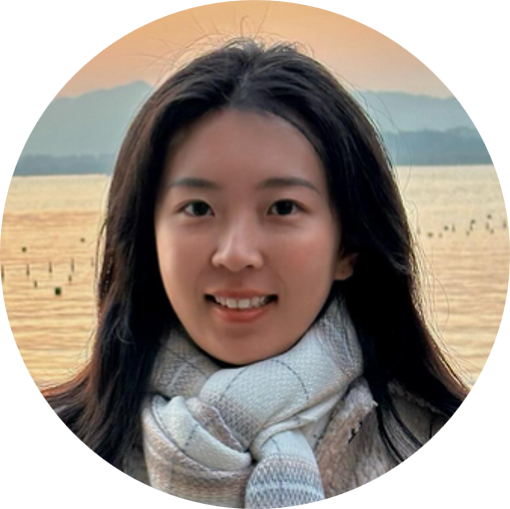
Paris Zhang
NVIDIA
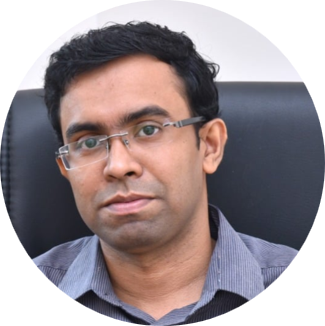
Pranamesh Chakraborty
Indian Institute of Technology Kanpur
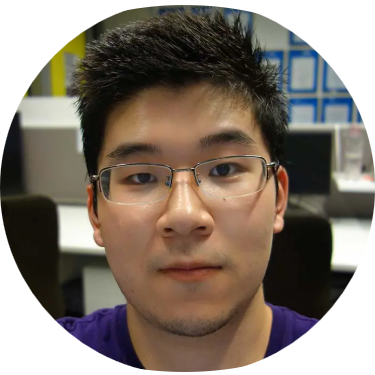
Zhedong Zheng
University of Macau
CITATIONS
Please cite the following papers accordingly if you choose to work with our datasets or refer to the previous challenge results:
2024 challenge summary paper – The 8th AI City Challenge
@InProceedings{Shuo24AIC24,
author = {Shuo Wang and David C. Anastasiu and Zheng Tang and Ming-Ching Chang and Yue Yao and Liang Zheng and Mohammed Shaiqur Rahman and Meenakshi S. Arya and Anuj Sharma and Pranamesh Chakraborty and Sanjita Prajapati and Quan Kong and Norimasa Kobori and Munkhjargal Gochoo and Munkh-Erdene Otgonbold and Ganzorig Batnasan and Fady Alnajjar and Ping-Yang Chen and Jun-Wei Hsieh and Xunlei Wu and Sameer Satish Pusegaonkar and Yizhou Wang and Sujit Biswas and Rama Chellappa},
title = {The 8th {AI City Challenge}},
booktitle = {The IEEE Conference on Computer Vision and Pattern Recognition (CVPR) Workshops},
month = {June},
year = {2024},
}
2023 challenge summary paper – The 7th AI City Challenge
@InProceedings{Naphade23AIC23,
author = {Milind Naphade and Shuo Wang and David C. Anastasiu and Zheng Tang and Ming-Ching Chang and Yue Yao and Liang Zheng and Mohammed Shaiqur Rahman and Meenakshi S. Arya and Anuj Sharma and Qi Feng and Vitaly Ablavsky and Stan Sclaroff and Pranamesh Chakraborty and Sanjita Prajapati and Alice Li and Shangru Li and Krishna Kunadharaju and Shenxin Jiang and Rama Chellappa},
title = {The 7th AI City Challenge},
booktitle = {The IEEE Conference on Computer Vision and Pattern Recognition (CVPR) Workshops},
month = {June},
year = {2023},
}
2022 challenge summary paper – The 6th AI City Challenge
@InProceedings{Naphade22AIC22,
author = {M. Naphade and S. Wang and D. C. Anastasiu and Z. Tang and M. Chang and Y. Yao and L. Zheng and M. Shaiqur Rahman and A. Venkatachalapathy and A. Sharma and Q. Feng and V. Ablavsky and S. Sclaroff and P. Chakraborty and A. Li and S. Li and R. Chellappa},
title = {The 6th AI City Challenge},
booktitle = {2022 IEEE/CVF Conference on Computer Vision and Pattern Recognition Workshops (CVPRW)},
month = {June},
year = {2022},
pages = {3346-3355},
doi = {10.1109/CVPRW56347.2022.00378},
publisher = {IEEE Computer Society}
}
2021 challenge summary paper – The 5th AI City Challenge
@InProceedings{Naphade21AIC21,
author = {Milind Naphade and Shuo Wang and David C. Anastasiu and Zheng Tang and Ming-Ching Chang and Xiaodong Yang and Yue Yao and Liang Zheng and Pranamesh Chakraborty and Christian E. Lopez and Anuj Sharma and Qi Feng and Vitaly Ablavsky and Stan Sclaroff},
title = {The 5th AI City Challenge},
booktitle = {The IEEE Conference on Computer Vision and Pattern Recognition (CVPR) Workshops},
month = {June},
year = {2021},
}
2020 challenge summary paper – The 4th AI City Challenge
@InProceedings{Naphade20AIC20,
author = {Milind Naphade and Shuo Wang and David C. Anastasiu and Zheng Tang and Ming-Ching Chang and Xiaodong Yang and Liang Zheng and Anuj Sharma and Rama Chellappa and Pranamesh Chakraborty},
title = {The 4th AI City Challenge},
booktitle = {The IEEE Conference on Computer Vision and Pattern Recognition (CVPR) Workshops},
month = {June},
year = {2020},
pages = {2665–2674}
}
2019 challenge summary paper – The 2019 AI City Challenge
@InProceedings{Naphade19AIC19,
author = {Milind Naphade and Zheng Tang and Ming-Ching Chang and David C. Anastasiu and Anuj Sharma and Rama Chellappa and Shuo Wang and Pranamesh Chakraborty and Tingting Huang and Jenq-Neng Hwang and Siwei Lyu},
title = {The 2019 AI City Challenge},
booktitle = {The IEEE Conference on Computer Vision and Pattern Recognition (CVPR) Workshops},
month = {June},
year = {2019},
pages = {452–460}
}
2018 challenge summary paper – The 2018 AI City Challenge
@inproceedings{Naphade18AIC18,
author={Milind Naphade and Ming-Ching Chang and Anuj Sharma and David C. Anastasiu and Vamsi Jagarlamudi and Pranamesh Chakraborty and Tingting Huang and Shuo Wang and Ming-Yu Liu and Rama Chellappa and Jenq-Neng Hwang and Siwei Lyu},
title = {The 2018 NVIDIA AI City Challenge},
booktitle = {Proc. CVPR Workshops},
pages = {53-–60},
year = 2018
}
2017 challenge summary paper – The NVIDIA AI City Challenge
@inproceedings{Naphade17AIC17,
author={Milind Naphade and David C. Anastasiu and Anuj Sharma and Vamsi Jagrlamudi and Hyeran Jeon and Kaikai Liu and Ming-Ching Chang and Siwei Lyu and Zeyu Gao},
title={The NVIDIA AI City Challenge},
booktitle = {Prof. SmartWorld},
address = {Santa Clara, CA, USA},
year = 2017
}
Woven Traffic Safety (WTS) Dataset
@article{WTS2024,
title = {WTS: A Pedestrian-Centric Traffic Video Dataset for Fine-grained Spatial-Temporal Understanding},
author = {Quan Kong and Yuki Kawana and Rajat Saini and Ashutosh Kumar and Jingjing Pan and Ta Gu and Yohei Ozao and Balazs Opra and David C. Anastasiu and Yoichi Sato and Norimasa Kobori},
year = {2024},
url = {https://github.com/woven-visionai/wts-dataset}
}
Synthetic Distracted Driving (SynDD2) dataset: Synthetic Distracted Driving (SynDD2) dataset for analyzing distracted behaviors and various gaze zones of a driver
@InProceedings{rahman2023synthetic,
author={Mohammed Shaiqur Rahman and Jiyang Wang and Senem Velipasalar Gursoy and David Anastasiu and Shuo Wang and Anuj Sharma},
title = {Synthetic Distracted Driving (SynDD2) dataset for analyzing distracted behaviors and various gaze zones of a driver},
howpublished = {arXiv:2204.08096},
year = {2023}
}
Synthetic distracted driving (SynDD1) dataset: Synthetic distracted driving (SynDD1) dataset for analyzing distracted behaviors and various gaze zones of a driver
@InProceedings{RAHMAN2023108793,
author={Mohammed Shaiqur Rahman and Archana Venkatachalapathy and Anuj Sharma and Jiyang Wang and Senem Velipasalar Gursoy and David Anastasiu and Shuo Wang},
title = {Synthetic distracted driving (SynDD1) dataset for analyzing distracted behaviors and various gaze zones of a driver},
journal = {Data in Brief},
volume = {46},
pages = {108793},
year = {2023},
issn = {2352-3409},
doi = {https://doi.org/10.1016/j.dib.2022.108793}
}
Natural language-based vehicle retrieval dataset: CityFlow-NL: Tracking and Retrieval of Vehicles at City Scale by Natural Language Descriptions
@InProceedings{Feng21CityFlowNL,
author={Qi Feng and Vitaly Ablavsky and Stan Sclaroff},
title = {CityFlow-NL: Tracking and Retrieval of Vehicles at City Scale by Natural Language Descriptions},
howpublished = {arXiv:2101.04741},
year = {2021}
}
Vehicle MTMC tracking & re-identification dataset – CityFlow: A City-Scale Benchmark for Multi-Target Multi-Camera Vehicle Tracking and Re-Identification
@InProceedings{Tang19CityFlow,
author = {Zheng Tang and Milind Naphade and Ming-Yu Liu and Xiaodong Yang and Stan Birchfield and Shuo Wang and Ratnesh Kumar and David Anastasiu and Jenq-Neng Hwang},
title = {CityFlow: A City-Scale Benchmark for Multi-Target Multi-Camera Vehicle Tracking and Re-Identification},
booktitle = {The IEEE Conference on Computer Vision and Pattern Recognition (CVPR)},
month = {June},
year = {2019},
pages = {8797–8806}
}
Synthetic 3D vehicle dataset – Simulating Content Consistent Vehicle Datasets with Attribute Descent
@InProceedings{Yao20VehicleX,
author={Yue Yao and Liang Zheng and Xiaodong Yang and Milind Naphade and Tom Gedeon},
title = {Simulating Content Consistent Vehicle Datasets with Attribute Descent},
booktitle = {The European Conference on Computer Vision (ECCV)},
month = {August},
year = {2020},
pages = {775–791}
}