2021 AI CITY CHALLENGE
Transportation is one of the largest segments that can benefit from actionable insights derived from data captured by sensors. Between traffic, signaling systems, transportation systems, infrastructure, and transit, the opportunity for insights from these sensors to make transportation systems smarter is immense. Unfortunately, there are several reasons why these potential benefits have not yet materialized. Poor data quality, the lack of labels for the data, and the lack of high-quality models that can convert the data into actionable insights are some of the biggest impediments to unlocking the value of the data. There is a need for platforms that allow for appropriate analysis from edge to cloud, which will accelerate the development and deployment of these models. The AI City Challenge Workshop at CVPR 2021 will specifically focus on ITS problems such as:
- Turn-counts used by DOTs for signal timing planning
- City-scale multi-camera vehicle re-identification w. real and synthetic training data
- City-scale multi-camera vehicle tracking
- Anomaly detection – detecting anomalies such as crashes, stalled vehicles, etc.
- Natural language-based vehicle track retrieval
We solicit original contributions in these and related areas where computer vision, natural language processing, and specifically deep learning have shown promise in achieving large scale practical deployment that will help make cities smarter.
To accelerate the research and development of techniques, the 5th edition of this Challenge will push the research and development in several new ways. First, the challenge will introduce a new track for multi-camera retrieval of vehicle trajectories based on natural language descriptions of the targets. To our knowledge, this will be the first such challenge that combines computer vision and natural language processing for city-scale retrieval implementations needed by DOTs for operational deployments of these systems. The second change in this edition will be the expansion of training and testing sets in several challenge tracks, which prevents participating teams from reusing models that have already saturated the performance on the previous test sets. Finally, our vehicle counting track will now require online, rather than batch, algorithms that must efficiently run on IoT devices, which further pushes the envelope of what is possible and brings the technology closer to integration into DOT systems.
Important Dates
-
Challenge kick-off: Tuesday, Jan 12 -
Data sets shared with participants: Friday, Jan 22 -
Evaluation server open to submissions: Saturday, March 6 -
Challenge track submissions due: Friday, April 9 (11:59 PM, Pacific Time)
Evaluation submission is closed and rankings are finalized. -
Workshop papers due: Tuesday, April 13 (09:00 AM, Pacific Time)
Since our review is not double-blind, papers should be submitted in final/camera-ready form. -
Final decisions to authors: Sunday, April 18
All authors are notified in CMT. There are about 24 hours to prepare for the final version of accepted papers. -
Final papers due: Monday, April 19 (11:59 PM, Pacific Time)
All camera ready paper should be uploaded to CMT to be published by CVPR 2021. The accepted workshop papers will be accessible online at IEEE Xplore Digital Library and CVF Open Access. -
Open source on GitHub (training code + testing code + additional annotation) due: Sunday, May 9 (11:59 PM, Pacific Time)
All the competitors/candidates for awards MUST release their code for validation before decision of awardees. The performance on the leaderboard has to be reproducible without the use of external data. -
Presentation of papers and announcement of awards: Friday, June 25 (02:00 PM, Pacific Time)
ORGANIZING COMMITTEE
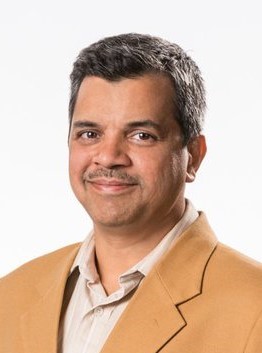
Milind Naphade
NVIDIA Corporation
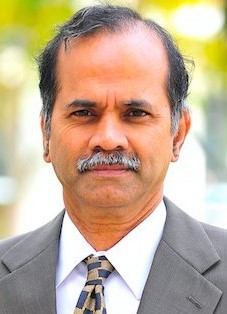
Rama Chellappa
Johns Hopkins University
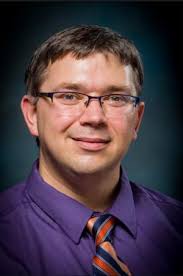
David Anastasiu
Santa Clara University
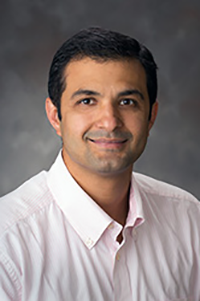
Anuj Sharma
Iowa State University
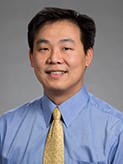
Ming-Ching Chang
University at Albany – SUNY
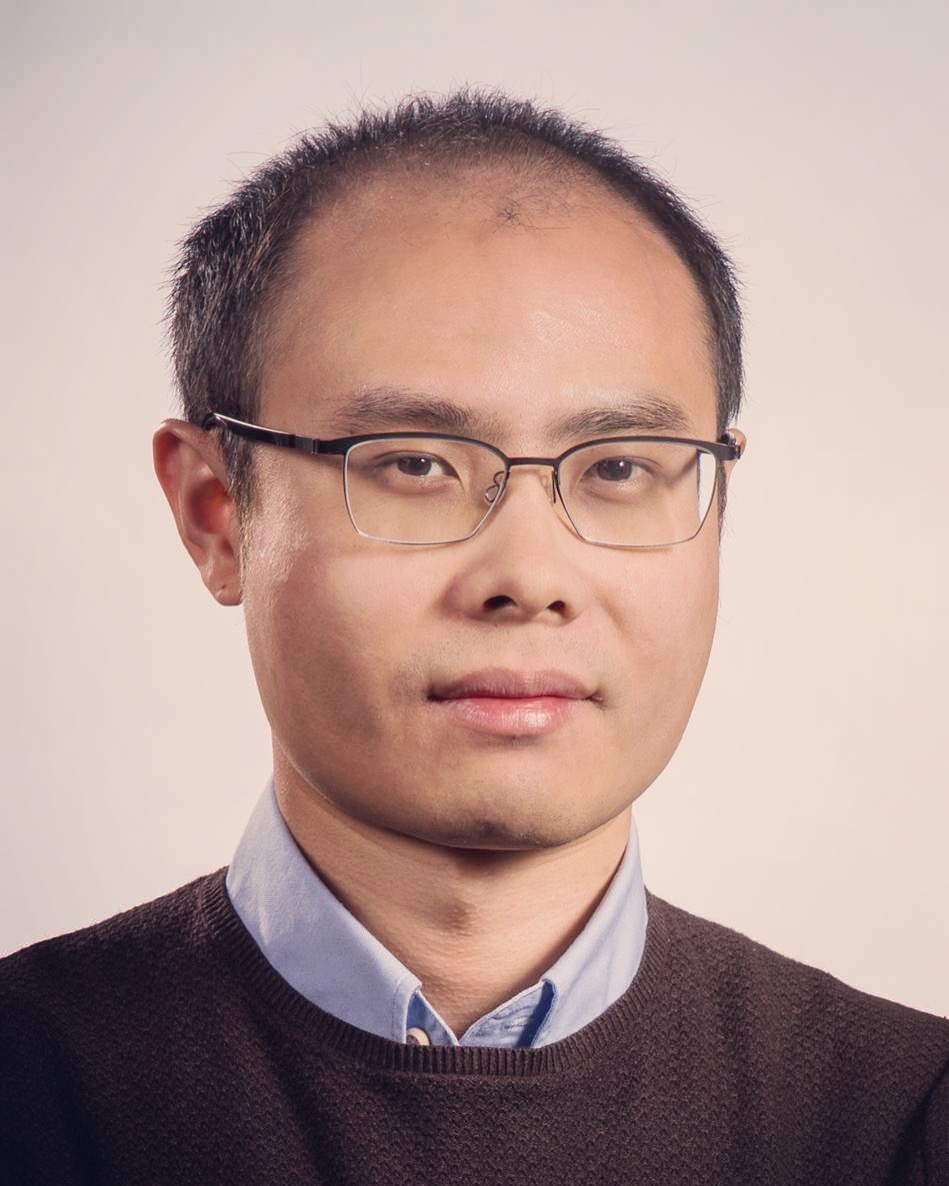
Xiaodong Yang
QCraft
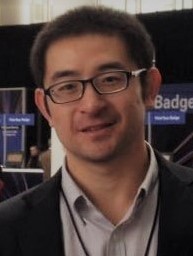
Shuo Wang
NVIDIA Corporation
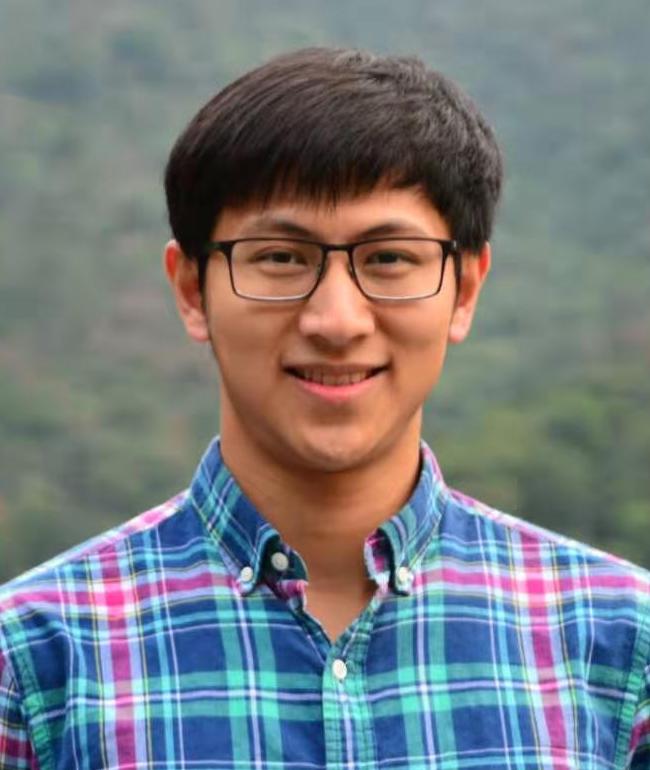
Zheng Tang
NVIDIA Corporation
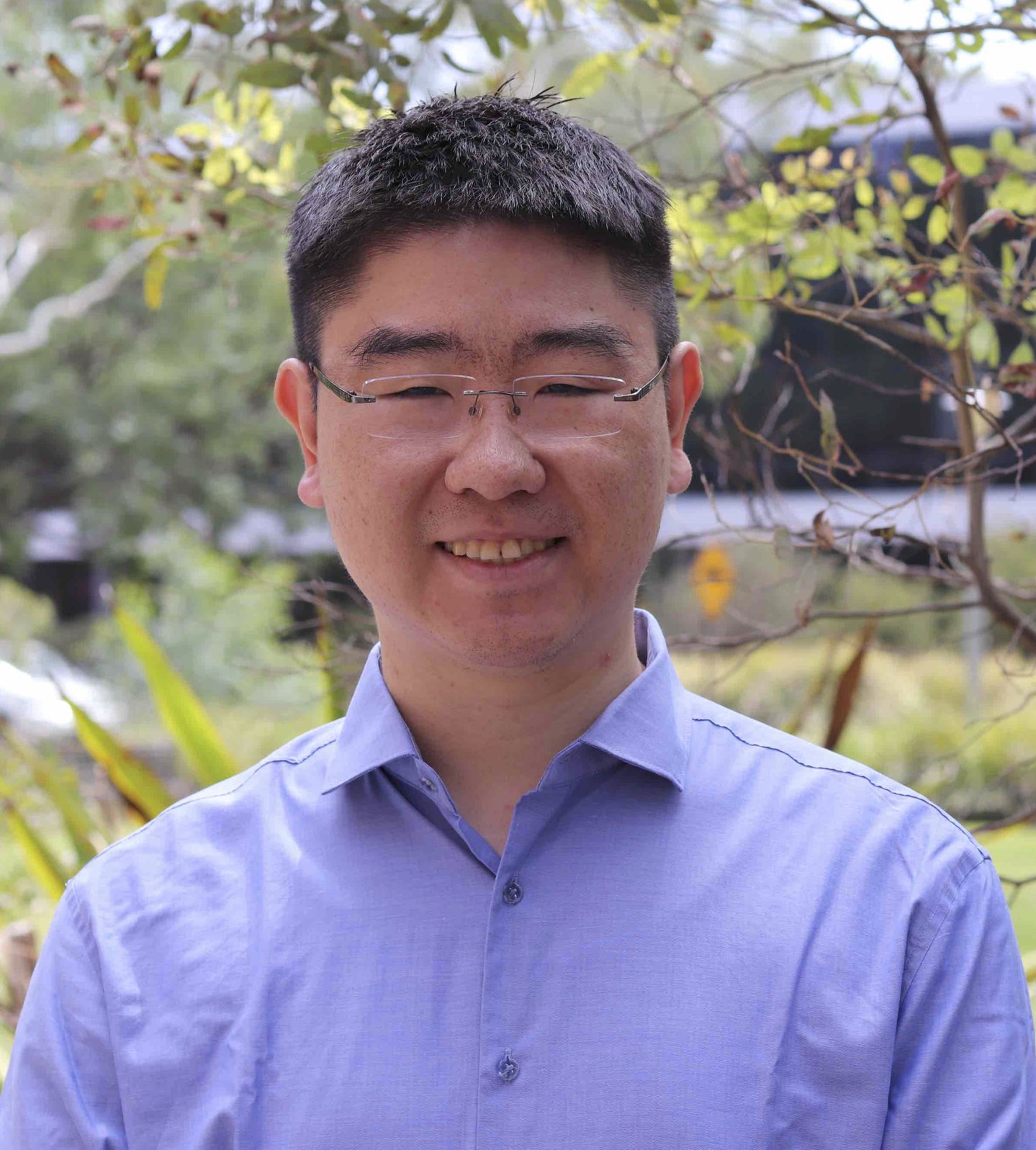
Liang Zheng
Australian National University
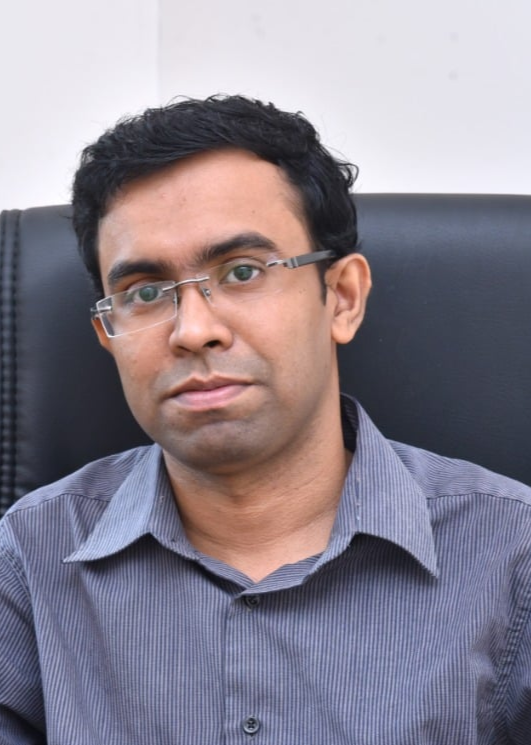
Pranamesh Chakraborty
Indian Institute of Technology Kanpur
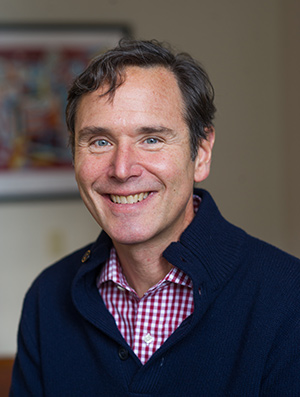
Stan Sclaroff
Boston University
CITATIONS
Please cite the following papers accordingly if you choose to work with our datasets or refer to the previous challenge results:
2021 challenge summary paper – The 5th AI City Challenge
@InProceedings{Naphade21AIC21,
author = {Milind Naphade and Shuo Wang and David C. Anastasiu and Zheng Tang and Ming-Ching Chang and Xiaodong Yang and Yue Yao and Liang Zheng and Pranamesh Chakraborty and Christian E. Lopez and Anuj Sharma and Qi Feng and Vitaly Ablavsky and Stan Sclaroff},
title = {The 5th AI City Challenge},
booktitle = {The IEEE Conference on Computer Vision and Pattern Recognition (CVPR) Workshops},
month = {June},
year = {2021},
}
2020 challenge summary paper – The 4th AI City Challenge
@InProceedings{Naphade20AIC20,
author = {Milind Naphade and Shuo Wang and David C. Anastasiu and Zheng Tang and Ming-Ching Chang and Xiaodong Yang and Liang Zheng and Anuj Sharma and Rama Chellappa and Pranamesh Chakraborty},
title = {The 4th AI City Challenge},
booktitle = {The IEEE Conference on Computer Vision and Pattern Recognition (CVPR) Workshops},
month = {June},
year = {2020},
pages = {2665–2674}
}
2019 challenge summary paper – The 2019 AI City Challenge
@InProceedings{Naphade19AIC19,
author = {Milind Naphade and Zheng Tang and Ming-Ching Chang and David C. Anastasiu and Anuj Sharma and Rama Chellappa and Shuo Wang and Pranamesh Chakraborty and Tingting Huang and Jenq-Neng Hwang and Siwei Lyu},
title = {The 2019 AI City Challenge},
booktitle = {The IEEE Conference on Computer Vision and Pattern Recognition (CVPR) Workshops},
month = {June},
year = {2019},
pages = {452–460}
}
2018 challenge summary paper – The 2018 AI City Challenge
@inproceedings{Naphade18AIC18,
author={Milind Naphade and Ming-Ching Chang and Anuj Sharma and David C. Anastasiu and Vamsi Jagarlamudi and Pranamesh Chakraborty and Tingting Huang and Shuo Wang and Ming-Yu Liu and Rama Chellappa and Jenq-Neng Hwang and Siwei Lyu},
title = {The 2018 NVIDIA AI City Challenge},
booktitle = {Proc. CVPR Workshops},
pages = {53-–60},
year = 2018
}
2017 challenge summary paper – The NVIDIA AI City Challenge
@inproceedings{Naphade17AIC17,
author={Milind Naphade and David C. Anastasiu and Anuj Sharma and Vamsi Jagrlamudi and Hyeran Jeon and Kaikai Liu and Ming-Ching Chang and Siwei Lyu and Zeyu Gao},
title={The NVIDIA AI City Challenge},
booktitle = {Prof. SmartWorld},
address = {Santa Clara, CA, USA},
year = 2017
}
Natural language-based vehicle retrieval dataset: CityFlow-NL: Tracking and Retrieval of Vehicles at City Scale by Natural Language Descriptions
@InProceedings{Feng21CityFlowNL,
author={Qi Feng and Vitaly Ablavsky and Stan Sclaroff},
title = {CityFlow-NL: Tracking and Retrieval of Vehicles at City Scale by Natural Language Descriptions},
howpublished = {arXiv:2101.04741},
year = {2021}
}
Vehicle MTMC tracking & re-identification dataset – CityFlow: A City-Scale Benchmark for Multi-Target Multi-Camera Vehicle Tracking and Re-Identification
@InProceedings{Tang19CityFlow,
author = {Zheng Tang and Milind Naphade and Ming-Yu Liu and Xiaodong Yang and Stan Birchfield and Shuo Wang and Ratnesh Kumar and David Anastasiu and Jenq-Neng Hwang},
title = {CityFlow: A City-Scale Benchmark for Multi-Target Multi-Camera Vehicle Tracking and Re-Identification},
booktitle = {The IEEE Conference on Computer Vision and Pattern Recognition (CVPR)},
month = {June},
year = {2019},
pages = {8797–8806}
}
Synthetic 3D vehicle dataset – Simulating Content Consistent Vehicle Datasets with Attribute Descent
@InProceedings{Yao20VehicleX,
author={Yue Yao and Liang Zheng and Xiaodong Yang and Milind Naphade and Tom Gedeon},
title = {Simulating Content Consistent Vehicle Datasets with Attribute Descent},
booktitle = {The European Conference on Computer Vision (ECCV)},
month = {August},
year = {2020},
pages = {775–791}
}